AI Claims Adjudication: The Future of Healthcare in 2025
AI claims adjudication is revolutionizing healthcare in 2025 with faster, smarter, and more accurate claims processing.
4/17/20254 min read
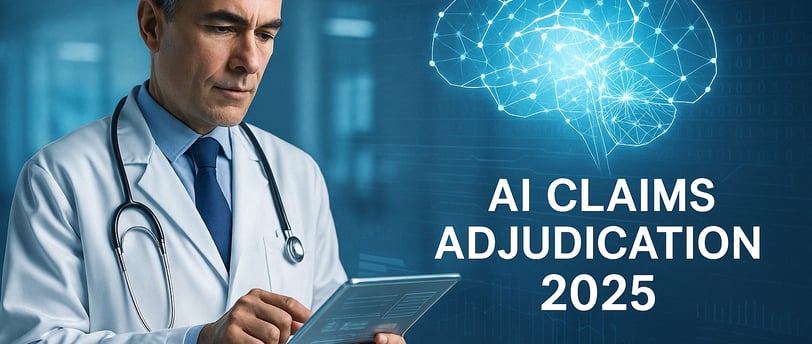
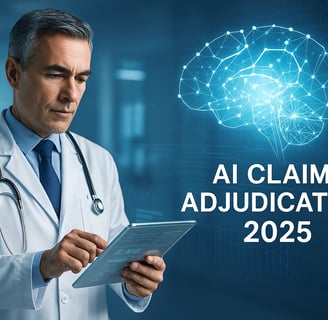
In 2025, the healthcare industry faces a paradox—rapid technological advancement on one hand, and a still-bloated, error-prone billing ecosystem on the other. As someone who has implemented and optimized AI-driven adjudication systems across multiple health systems and payer networks, I can say this with confidence:
“The future of claims adjudication is not just automated—it’s intelligent, context-aware, and self-improving.”
Let me walk you through the real impact of AI in claims adjudication—beyond the buzzwords, into the trenches of healthcare revenue cycle transformation.
What Is AI Claims Adjudication?
Claims adjudication has always been the heart of healthcare revenue integrity. But traditionally, it’s been a heart with an irregular beat—delayed payments, inconsistent policies, and mountains of manual work.
AI flips that on its head.
In my work with hospital systems and third-party administrators, we’ve deployed AI to:
Auto-validate codes against payer-specific rules in milliseconds
Apply NLP models to interpret unstructured data like physician notes and radiology reports
Trigger real-time denials management with suggested resolutions built in
Seamlessly escalate edge cases to human adjudicators with context-rich summaries
The magic? These systems learn over time. They analyze what led to denials, appeals, and payment success—and fine-tune themselves accordingly.
How AI Improves the Claims Adjudication Process
I’ve overseen dozens of AI deployments. Here’s what actually works when AI is implemented correctly in claims adjudication:
Pre-Adjudication Checks: AI scans documentation for incomplete fields or common coding inconsistencies before submission. This alone reduces denial rates by 25–40%.
Smart Policy Engines: We’ve embedded payer-specific rules directly into the AI logic—so if a payer doesn’t reimburse for a procedure with a certain diagnosis code, the claim is corrected automatically before submission.
Automated Medical Necessity Validation: AI checks the service against coverage policies using NLP-based summarization of medical notes. One payer we worked with reduced false denials by 60% using this method.
Exception Routing: Instead of routing everything to human review, only 8–15% of complex cases are flagged for further inspection—freeing up adjudicators to focus on true edge cases.
This isn’t future-state—it’s happening right now in revenue cycle departments that lean into smart automation.
Benefits of AI in Claims Adjudication
When AI is implemented with human-centric design in mind, the results are dramatic. Here’s what we consistently see in real-life deployments:
🕒 Turnaround Time Reduction
At one major payer, AI reduced the average adjudication cycle from 18 days to under 6 days—without increasing errors.
🧾 Payment Accuracy
We deployed an AI system that used contextual claims history to validate charges. It reduced overpayment rates by 30%, and decreased appeals by 45%.
🤖 Less Burnout, Better Staff Allocation
Your claims analysts shouldn’t be stuck keying in data—they should be resolving escalated exceptions and managing provider relationships. AI offloads 60–80% of the repetitive work.
💰 Real Revenue Gains
A Texas-based health system saw $12 million in recovered reimbursements within 12 months by combining AI adjudication with smart denial resolution workflows.
The ROI isn’t just in saved hours—it’s in recovered revenue, happier staff, and better payer-provider relationships.
Real-World Use Cases and Success Stories
Let’s get specific. Here’s what this looks like in action:
Mid-sized hospital group (Florida): Used Olive AI to automate coding and claims submission for radiology. Result: 3.6 FTEs worth of labor saved, 2.4x faster cycle time.
National payer (Confidential): Used AI to scan inpatient claims and predict fraud risk. Result: reduced overpayments by 22%, caught 37% more suspicious claims before payment.
Federally Qualified Health Center (FQHC): Adopted an AI-first EHR and claims tool. Result: went from 78% clean claims rate to 95% in 6 months.
If you're not seeing results like this, chances are your AI tool is either rule-based (not truly intelligent) or lacks integration into the full RCM lifecycle.
Challenges and Limitations of AI in Adjudication
Let’s be honest—AI isn’t a magic wand. Here are the top five challenges I’ve personally seen, and how we tackled them:
1. Data Quality and Integrity
Garbage in, garbage out. AI needs clean data. We invested in EHR integration and used middleware to structure unstructured clinical content before feeding it into the model.
2. Black Box Risk
Providers get nervous when they don’t understand why a claim is denied. That’s why we prioritized explainable AI (XAI)—adding clear audit trails and human-readable justifications for each AI decision.
3. Staff Adoption
Billing teams often fear automation. We solved this by positioning AI as an assistant, not a replacement—and training staff to use AI to flag issues and reduce their own errors.
4. Payer Variability
Every payer has different rules. We built modular “payer logic packs” that let AI systems plug in different adjudication rules based on the destination.
5. Oversight and Compliance
You must involve compliance teams early. We ensured our AI systems log every action and follow strict HIPAA, HITRUST, and CMS audit protocols.
The Future Outlook for AI Claims Adjudication
In 2025 and beyond, here’s where I see things headed based on trends and ongoing client work:
Self-healing Workflows: AI systems that fix claim issues before they become denials—without human intervention
Generative AI in Appeals: LLMs that write personalized, evidence-backed appeal letters in seconds
Real-time Interoperability: Cloud-native systems where payers and providers collaborate live on claims status and resolutions
Full-cycle AI: From patient intake to billing to collections—AI managing the entire RCM ecosystem
AI-Powered Provider Portals: Tools that proactively guide providers on what’s needed to ensure clean claims the first time
We’re not just automating what we’ve always done—we’re building something smarter, faster, and fundamentally more fair for providers and patients alike.
Conclusion
AI claims adjudication in 2025 isn’t science fiction—it’s clinical, operational, and financial transformation in real time.
I’ve seen firsthand how smart, well-integrated AI systems can:
Slash processing time
Increase clean claim rates
Minimize burnout
Drive millions in recoverable revenue
But it only works if implemented with precision, compliance, and collaboration in mind.
If you’re still relying on paper-based processes, fragmented systems, or outdated rules engines—it’s time to rethink your approach.
📣 Start now. Evaluate your current RCM tech. Talk to vendors with proven case studies. Most importantly, treat AI as a partner in adjudication, not a disruptor.
Let’s build a smarter revenue cycle—together.
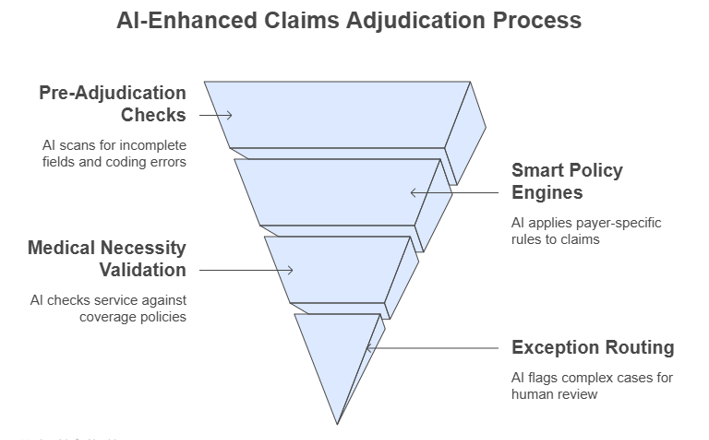
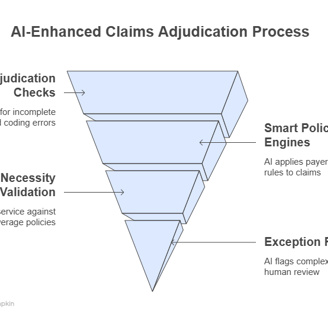
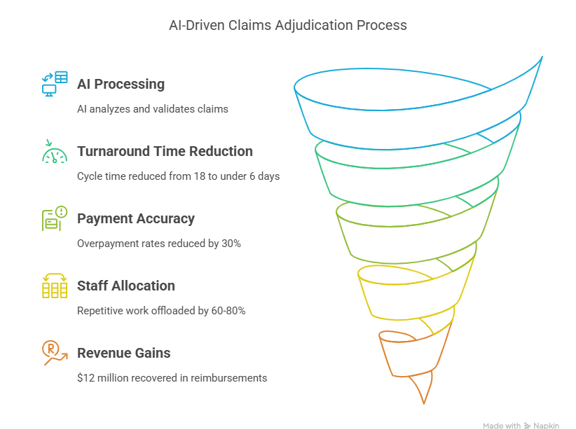
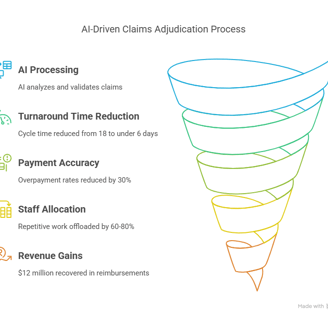
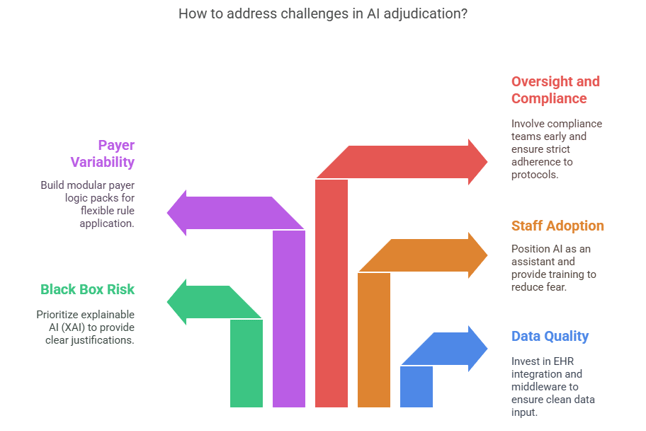
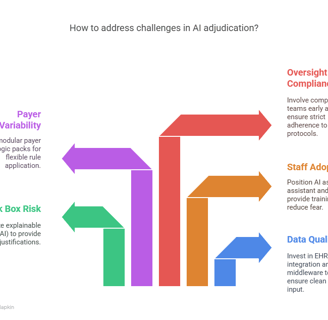