NLP in Medical Coding: Transforming Healthcare in 2025
Explore how NLP is revolutionizing medical coding in 2025—automating processes, reducing errors, and improving healthcare delivery.
4/15/20254 min read
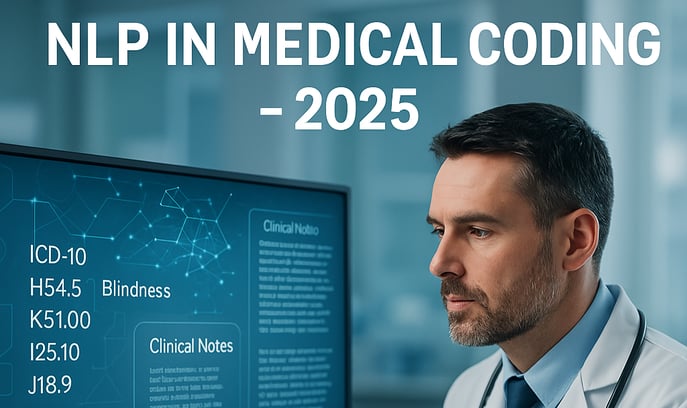
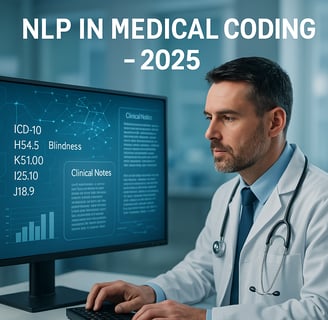
Introduction
“80% of healthcare data is unstructured—yet it holds the answers to better care, faster reimbursement, and improved outcomes.” – IBM Watson Health
In 2025, healthcare providers are under more pressure than ever. Reimbursement regulations are tighter, clinician burnout is at an all-time high, and the complexity of medical documentation is growing. Amid these challenges, Natural Language Processing (NLP) has emerged as a game-changer.
As someone deeply involved in the AI-healthcare intersection, I can confidently say that NLP isn’t just another buzzword—it’s a strategic necessity. It's helping health systems convert oceans of unstructured data (clinical notes, radiology reports, operative summaries) into structured, billable codes—at scale and with accuracy.
Whether you're a coder, provider, compliance officer, or tech leader, this article is your guide to understanding where NLP in medical coding stands today, where it’s headed, and how to make it work for your organization.
What Is Natural Language Processing (NLP) in Healthcare?
NLP is a subfield of AI that allows computers to process and understand human language. In healthcare, it serves a specialized function: extracting actionable data from messy, free-text clinical documentation.
From a technical standpoint:
Named Entity Recognition (NER) identifies concepts like diseases, medications, and procedures.
Contextual Analysis helps distinguish negation (“no signs of stroke”) from affirmation (“history of stroke”).
Normalization maps recognized entities to standardized vocabularies like SNOMED CT, LOINC, or ICD-10.
In short, NLP turns messy clinician notes into code-ready, structured information.
Key 2025 Vendors Leading in NLP for Coding:
3M™ M*Modal Fluency for Coding: One of the most widely adopted CAC systems.
Clinithink’s CLiX Enrich: Known for its deep NLP capabilities and integration with HL7/FHIR APIs.
Amazon Comprehend Medical: Cloud-based NLP API with strong scalability and PHI redaction tools.
NVIDIA Clara Holoscan + MONAI: Bringing real-time NLP to radiology and surgical environments.
The Medical Coding Process Explained
Medical coding serves as the foundation for healthcare reimbursement and analytics. Traditionally:
Clinicians document patient encounters in narrative format.
Human coders review this documentation and assign ICD-10-CM (diagnoses), CPT (procedures), or HCPCS (supplies and services) codes.
These codes are submitted to payers (insurance, CMS) for reimbursement.
Challenges in 2025:
Clinical notes are longer due to regulatory documentation demands.
Coders face higher pressure to maintain accuracy under tight timelines.
Remote and hybrid work models require better digital infrastructure for secure, efficient workflows.
NLP systems reduce this burden by pre-populating code suggestions, surfacing documentation gaps, and even providing compliance hints during documentation.
How NLP Enhances Medical Coding Accuracy
Here’s where the true value of NLP kicks in—contextual understanding and precision.
Let’s say a provider notes:
“Patient with suspected pneumonia, awaiting radiology confirmation. Started empiric antibiotics.”
Traditional systems might code this as pneumonia. A well-trained NLP engine recognizes “suspected” and avoids overcoding, flagging it as queryable or inconclusive.
What NLP Gets Right in 2025:
Negation detection (e.g., “no evidence of fracture”).
Temporal sequencing (e.g., “history of asthma, resolved in 2020”).
Uncertainty indicators (e.g., “likely,” “possibly,” “considering”).
Anatomic relationships (e.g., “mass in right upper quadrant” vs. “no mass in RUQ”).
In environments like ERs and surgical suites where turnaround time is critical, coding accuracy improvements of 30–50% have been documented when using NLP-assisted tools.
Use Cases and Real-World Applications
The last two years have seen massive adoption of NLP across leading health systems.
Real-World Examples:
Kaiser Permanente: Uses NLP to auto-classify problem lists and suggest billing codes within Epic.
UCLA Health: Applies NLP for automated tumor registry data abstraction—saving 500+ hours per month.
Mount Sinai Health System: Leveraging NLP to improve clinical decision support (CDS) accuracy in Epic’s BestPractice Advisories.
Veterans Health Administration (VHA): Running large-scale pilots for NLP-based coding assistance for over 9 million veterans.
These organizations are not replacing coders—they’re reallocating them to high-value tasks like audit defense, DRG optimization, and compliance review
Benefits of Using NLP in Medical Coding
The benefits aren’t theoretical—they’re measurable and strategic:
✅ Revenue Cycle Acceleration
NLP reduces days in accounts receivable (A/R) by up to 18%.
Faster claim submissions = faster payments.
✅ Coder Productivity
1 coder can process 20–30% more records/day with NLP assistance.
AI handles the routine. Coders focus on edge cases.
✅ Fewer Denials
NLP tools reduce clinical documentation gaps that lead to medical necessity denials.
2024 surveys report a 35% reduction in first-pass denials after NLP deployment.
✅ Scalability and Flexibility
NLP works across inpatient, outpatient, radiology, pathology, and specialty practices.
Cloud-based NLP solutions enable multi-location deployment.
Challenges and Limitations of NLP in Medical Coding
No solution is perfect—especially when healthcare complexity is involved.
Common Limitations:
NLP can misinterpret ambiguous phrases without sufficient context (e.g., “negative for chest pain, cough”).
Training data quality is key—models trained on outdated or biased documentation won’t generalize well.
NLP struggles with nonstandard shorthand or heavily templated EMRs.
Explainability is critical for audits. Systems must show why a code was selected—just like a human coder.
Key Tip: Use NLP in tandem with human oversight (computer-assisted coding, or CAC), especially for high-risk coding categories like oncology, surgery, and mental health.
Compliance, Ethics, and AI Regulation
In 2025, CMS and ONC have updated their guidelines for AI transparency and auditability, requiring all AI-driven coding tools to:
Maintain audit trails of decision logic.
Be trainable using local institutional data.
Ensure no automated overcoding or DRG creep (intentional inflation of code severity).
Additionally:
NLP systems must comply with 42 CFR Part 2, HIPAA, and FHIR security standards.
Vendors are expected to offer explainable AI (XAI) dashboards that justify every suggestion.
Bottom line: AI in healthcare is now subject to the same scrutiny as human coders—and rightly so.
Future Trends: The Evolving Role of NLP in Healthcare Documentation
Conclusion
In 2025, Natural Language Processing is no longer optional for modern healthcare organizations—it’s foundational. From reducing denials to unlocking data buried in handwritten notes, NLP is quietly powering a smarter, faster, more compliant medical coding process.
But success lies in implementation. Choose the right vendor, prioritize explainability, and don’t ignore human oversight.
Want to future-proof your coding process? Start by piloting NLP in one department—track denial rates, coder throughput, and audit outcomes. The results may surprise you.
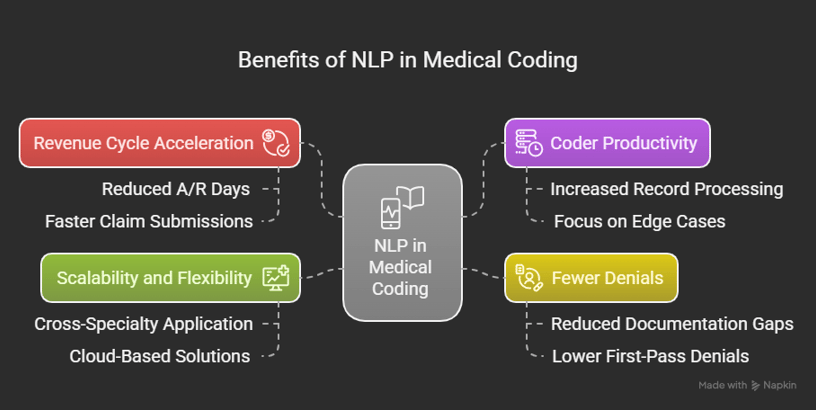
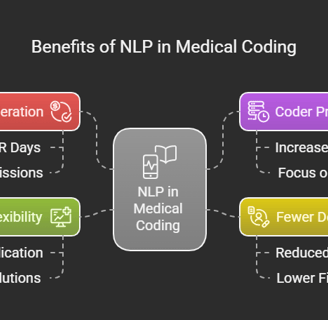
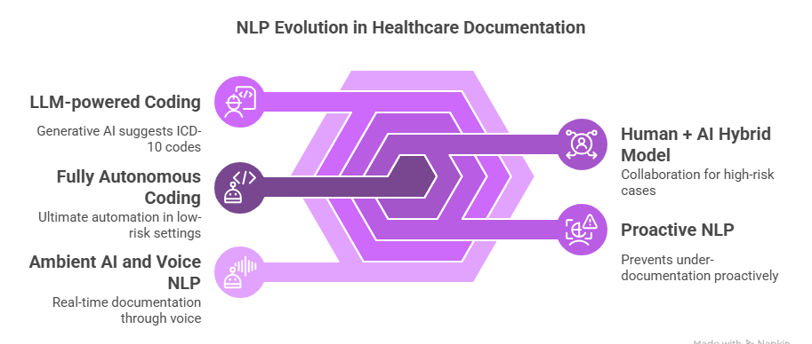
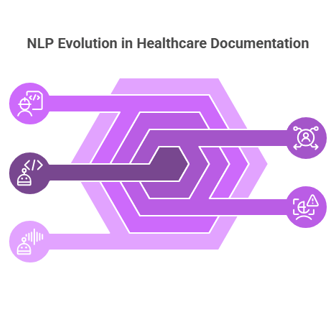